Customer Subscription Propensity Model
Media & Entertainment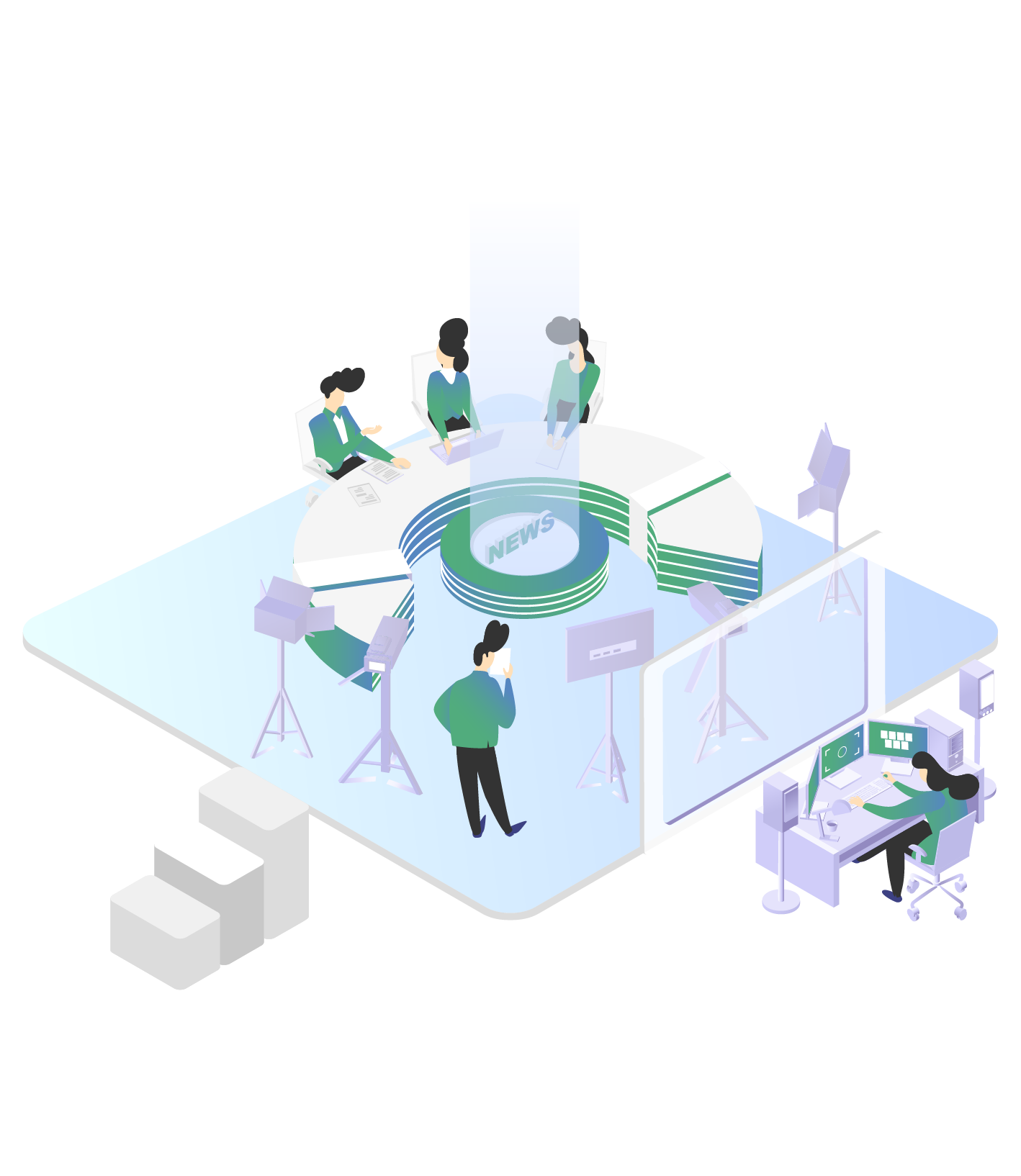
Business Impacts
Increase in Customer Conversion Rates
8 to 80%
Increase in F1 Score
Enhanced Return on Marketing Spend
Customer Key Facts
- Location : North America
- Industry : Media & Entertainment
Problem Context
The customer is one of the pioneers of the video streaming domain and a mobile video-on-demand service provider, with more than 7 million subscribers worldwide. Their OTT Platform offers both premium and free memberships. They wanted to develop a Machine Learning (ML) model that calculates the propensity of a user to upgrade to a premium subscription on their App.
Challenges
- Customer interaction data siloed across multiple platforms such as Amplitude, CleverTap, AppsFlyer, & other Ads platforms
- A low conversion rate led to a high imbalance in data
- Unavailability of historical data which dates back beyond 6 months
- Intrinsic predictability of user behavior was low
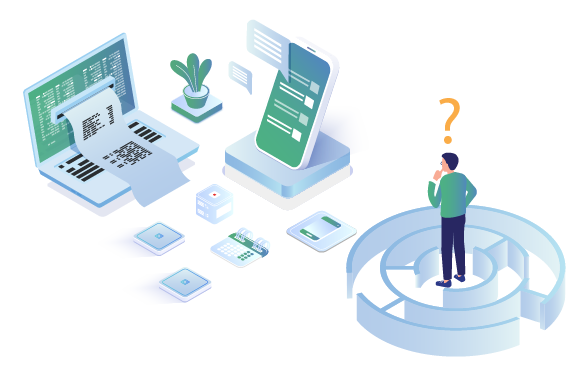
Technologies Used
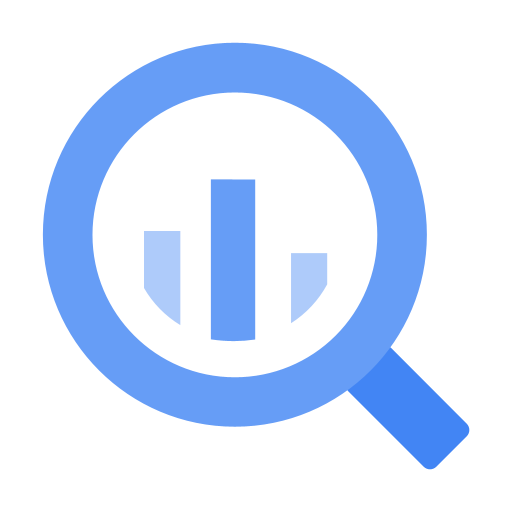
Google's BigQuery
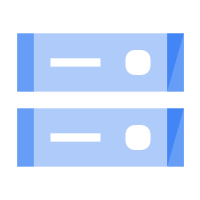
Google Cloud Storage
Google Cloud AutoML

Google Cloud Functions

Google Cloud Dataflow
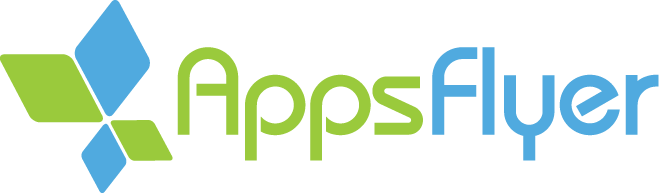
Appsflyer
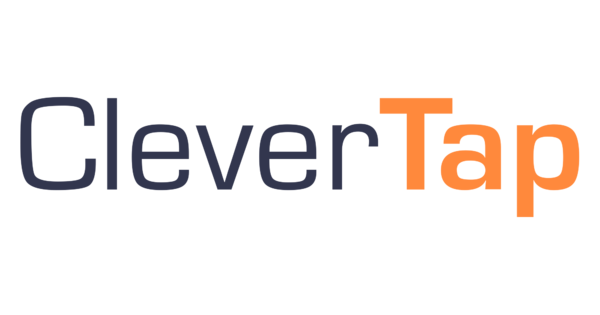
CleverTap
Identifying Customer Segments With High Conversion Probabilities
The customer already had an in-house subscription propensity model to predict the users who have a higher probability to upgrade to the premium membership. However, the model did not provide high accuracy.
Solution
Quantiphi built and trained a Subscription Propensity Model that provides customer segments with a higher probability of conversion for more targeted ad campaigns. First, data from various sources such as CleverTap, Amplitude, and AppsFlyer, was merged together in Google's BigQuery to form a unified user journey. Quantiphi performed EDA and feature engineering to identify the best sets of relevant attributes (i.e. high-impact features for conversion) to train the model. Within three weeks, more than 25 different ML modeling techniques were tested in order to overcome the data predictability challenge. As a result, the model could calculate the conversion probability scores for all the users who interacted with the App.
The best model results were achieved using Traditional ML Classifier. Once the model accuracy was improved, Quantiphi helped operationalize the solution to activate the audience on Google Ads by building a pipeline that sends the audience list from model output to Universal Ads Campaign for targeting.
Result
- Complete end-to-end automation of marketing funnel and dashboarding from multiple data sources
- A highly targeted audience set to re-market daily
- Higher subscription conversion rates
- A new stream of media spends for Google Ads from re-marketing
- Data-driven marketing leading to better ROI on marketing spends